Types of Predictive Models in AI & How to Create Them?
Let’s say you have just launched an eCommerce store. You see some organic visitors coming to your store and visiting a couple of pages. Over the period of time, not long though, you get your first checkout, but immediately there is a bounce off
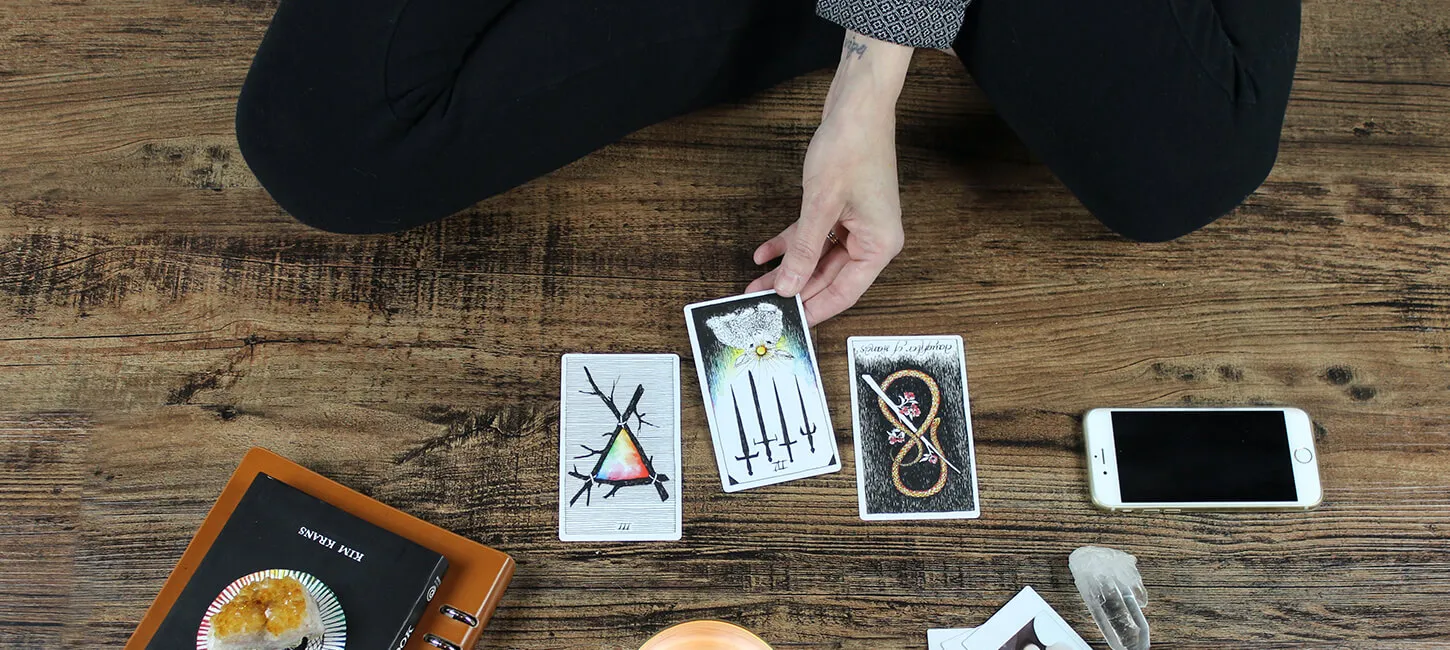
The same phenomenon happens again, and again, and again. You know what is happening, but you do not understand why this is happening. Since you are at the budding phase in your eCommerce journey, the sane step is to retrospective over your lost chances of sales.
Predictive analytics in AI with diagnostic and descriptive analytics can take you to the roots of the underlying issue. For example, you will be able to analyze that there’s something wrong with your checkout page. Maybe the rates of your products are low, but you are charging a high shipping price. No matter what, predictive analytics will help you determine the typical pattern your prospects follow using historical data. This historical data is then put to the best use for forecasting future outcomes.
This is a typical instance of the power of predictive analytics. Numerous types of predictive models are available to help your business capitalize on the right kind of insight.
Do you have limited minutes to read this blog?
Follow our TL;DR section with top FAQs about predictive modelling techniques and types of predictive models.
Let’s go with FAQs and get summarized understanding about Predictive Modelling:
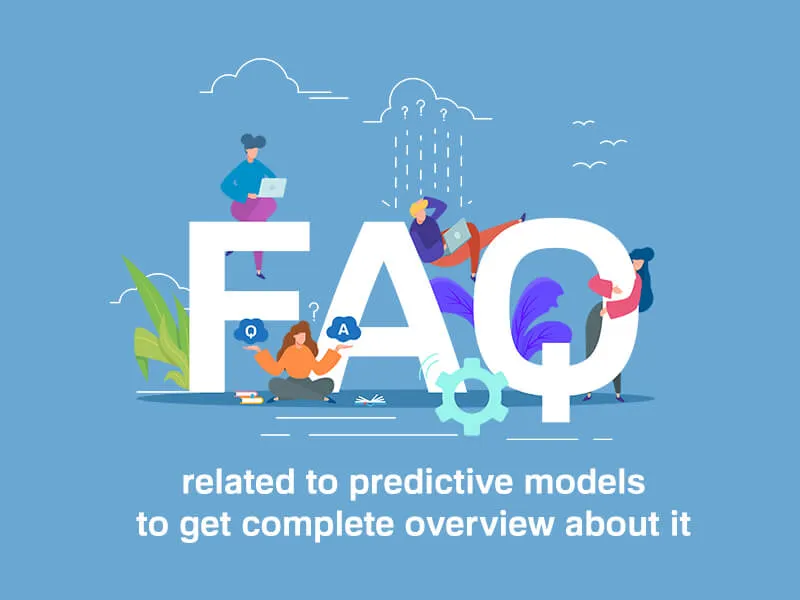
What is the Predictive Modelling Technique?
The predictive modelling technique is a definite process to predict future outcomes through a series of historical data. Therefore, the predictive analytical technique is a surefire way for a business to envision a solid future with actions indicated from the insights with high accuracy rates.
What is the Predictive Modelling Technique?
Below are some of the predictive modelling examples:
- Personalization of in-store experiences in retail stores.
- Predictive modelling for targeted advertising, messaging, and promotional offers.
- Assessing financial risk using predictive modelling in AI.
- Predictive modelling to thwart financial crime.
- Enabling banks and financial institutes to handle finances more securely with software.
- Curbing maintenance costs in aircraft using the data obtained from sensors about ageing and degradation of the parts of aircraft.
Can you Do Predictive Modelling in Excel?
Yes. It is possible to calculate predictive modelling in Excel. Below is the way to add an analysis tool pack to your Excel Workbook:
- Step 1: Files>>Options
- Step 2: Open Add-ins from the left side of the panel>>Manage Excel Add-ins>>Go.
- Step 3: Choose Analytics ToolPak and then press OK.
By following these steps, you will be able to get your analysis tool in your workbook for doing predictive modelling in Excel. If you want to check whether the tool has been added to Excel, you can do so by using the Data bar in the Ribbon.
What is Predictive Modelling Used for?
Predictive modelling can be used for different applications across industries such as manufacturing, sports, healthcare, eCommerce, finance, logistics, capital market, and more. Businesses can use predictive modelling to gain a competitive edge by gaining real-time data and insights through analytic tools. Predictive modelling is powerful enough to reveal any kind of risky activities for better predicting the maintenance needs of your business.
What is Predictive Modelling in Machine Learning?
Predictive modelling in machine learning is an analytical technique that uses machine learning as well as data mining for prediction and forecasting. With the use of predictive modelling in machine learning, business people can undertake sentiment analysis regarding the happiness quotient among their employees and customers.
What are Types of Predictive Models?
Find below the five crucial types of predictive models:
- Forecast model
- Classification model
- Outliers model
- Time series model
- Clustering model
Read more about these models in detail
What are the Benefits of Predictive Modelling Techniques?
Predictive modelling techniques leverage historical data for forecasting future outcomes. There are numerous statistical techniques such as data mining, predictive modelling, and machine learning.
Below are some key benefits of predictive modelling techniques:
- Identify opportunities for a new product or service
- Gain a competitive advantage
- Meet expectations of consumers
- Improve collaboration
- Get deeper insights about customers through their behavioural patterns
- Decrease risk and cost
- Optimize product as well as performance
- Avoid problems by addressing them before they occur
What are the Seven Steps of the Predictive Modelling Process?
In order to understand how predictive modelling processes work in actuality, below are the seven steps of the predictive modelling process:
- Know the real business intention
- Identify your predictive modelling goals
- Select data for predictive modelling
- Prepare data for analysis
- Transform the variables
- Select the right predictive modelling process
- Validate models through testing
Read the steps in detail in the section.
Similar to Predictive models, AI can be applied to any industry.
What are the Types of Predictive Models?
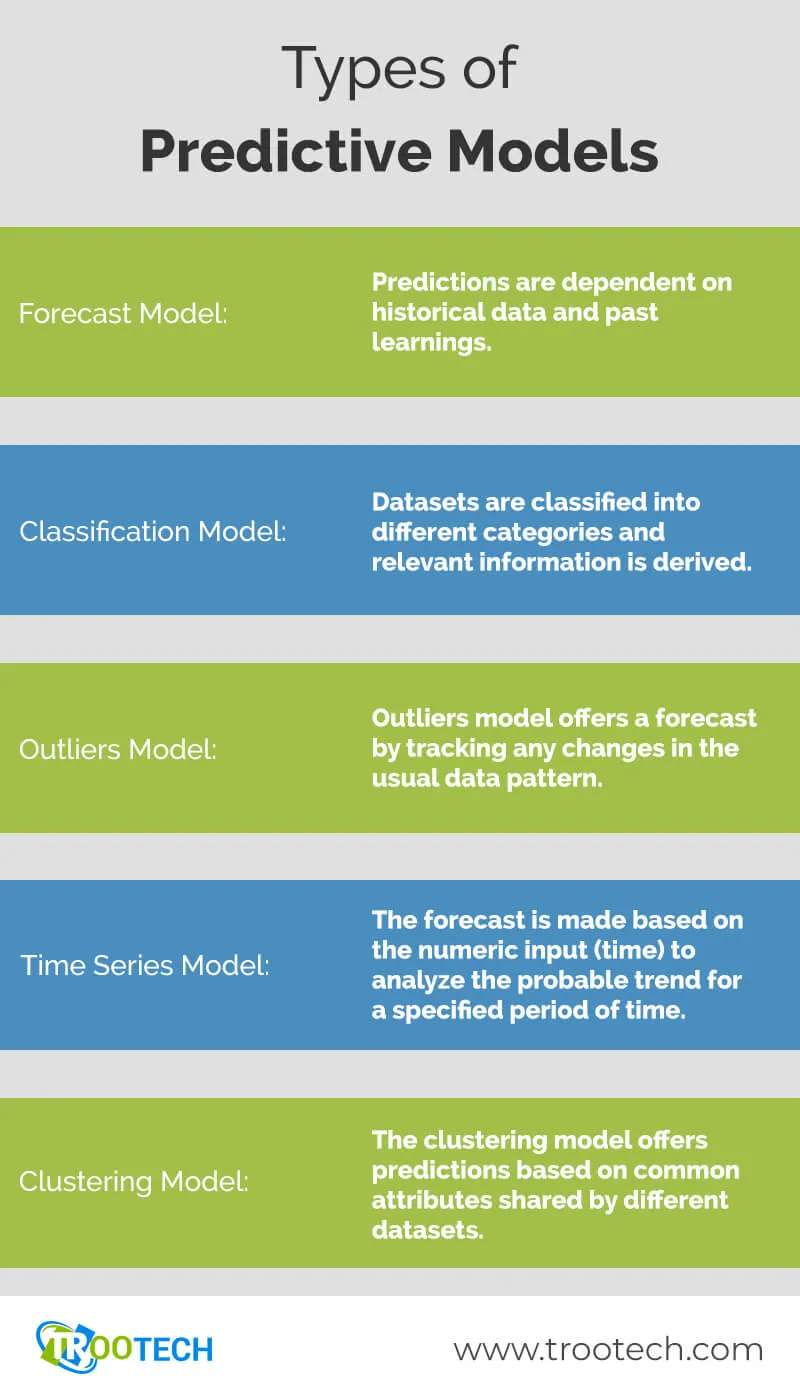
A predictive model is widely known as the subset of AI. It is not limited to predicting the future for your business, it can, in turn, propel growth and can be applied to numerous marketing as well as business scenarios.
There are multiple types of predictive models as well as algorithms. If you can figure out the right one for your business, you can make well-informed business decisions. Let’s take a look at each of these predictive models to understand them clearly. However, by no means this blog intends to restrict the types of predictive models to only five categories. We have curated and identified the most prominent predictive models:
Forecast Model
A forecast model manages the metric value prediction through the estimation of values of new data on the basis of learnings and insights from historical data. A forecast model is generally utilized to generate numerical values in historical data when none is available. One of the prominent strengths of the forecasting model is its capability to use multiple parameters as inputs. As a result, businesses usually prefer forecasting models. It operates through estimations of numeric values for new data based on the historical data.
Classification Model
As the name of the predictive model suggests, the classification model categorizes information on the basis of historical data. The classification predictive model can be applied to new and different industries as these can be retrained with new sets of data. As a result, the new data set can offer a wide analysis for answering questions. These classification models can be leveraged and applied to different industries such as finance and retail. Among the other types of predictive models, the classification model is one of the simplest techniques to get a ‘yes’ or ‘no’ category of answer.
Outliers Model
Now that we have seen how forecast and classification models interact with the historical data, the outlier predictive model works with anomalous data entries in a dataset. As per the name, anomalous data refers to the data that deviates from its usual pattern. The outlier predictive model works by tracking the unusual data, which are either with different numbers and categories or in isolation. This type of predictive model is highly useful in industries, wherein the identification of anomalies can safe-pocket millions of dollars from organizations by saving them from frauds and cyberthreats.
Time Series Model
The time series model of predictive analysis is focused on the data set, wherein the input parameter is time. The time series model executes through different data points for developing a numerical metric for predicting trends set for a specified period in time. If a business person wants to see how a particular element has changed over time, time-series analysis can be considered as a better predictive analytical model. One of the top areas that use time series models is the sales cycle. A small business owner wanting to track sales over time can rely on the time-series predictive model.
Clustering Model
The clustering model collects data and then categorizes it in the form of different groups on the basis of their common attributes. The ability to segregate data into various datasets, depending on particular attributes is useful for marketing applications. For instance, when a marketing executive wants to get information about the target audience, she can segregate a potential set of customer bases, depending on the common attributes. The clustering of data sets can be done in two ways–soft clustering and hard clustering. Hard clustering can categorize each of the data sets and identify whether a particular set is a part of a data cluster or not. On the contrary, soft clustering means allocating data probability while joining a data cluster.
Selecting the right model among the other types of predictive
Models can leave you confused. But TRooTech is your solution.
How to Develop Predictive Modelling Algorithm?
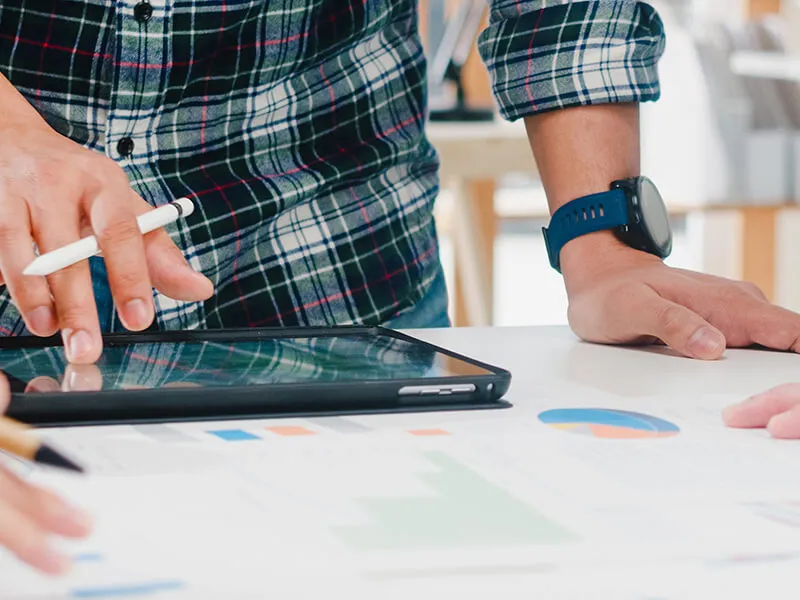
Predictive modelling leverages computational and mathematical methods for predicting an outcome. As you immerse in a certain project, there are certain milestones to take a close look at. The predictive model forecasts an outcome for a time-based or future-based state that changes the model input. These model parameters provide an explanation regarding the way model inputs influence the results.
Irrespective of the types of predictive models, the general process for developing a predictive modelling algorithm remains the same. Below are the key steps to creating a predictive modelling algorithm:
Know your real business intention:
When you start with a particular analysis, define the objectives first. The conclusion of the business model should be to address a burning question. When you have a clear objective in mind, it will enable you to define the scope of your project and offer the exact procedure to measure the success.
Identify your predictive modelling goals:
Based on the business question you need to answer, you can translate your objective into an analytical term. In order to identify your predictive modelling goals, you can take into account segmentation, profile analysis, risk modelling, response modelling, activation, attrition, cross-selling and upselling, customer lifetime value, and more.
Select data for predictive modelling
The next step is to select proper data for undertaking predictive modelling. In this step, you need to select the best data for target modelling by thoroughly examining and understanding your market, objective, and business. You can take into consideration the customer demographic, purchasing power, income, loyalty, transaction, macroeconomic factors, customer survey, market research, and competition.
Prepare data for analysis:
In order to get high-level accuracy in your analysis, you need to prepare the right set of data by taking the appropriate steps. You need to initially clean up your data for redundancy. The next step is to define variables as well as create a data dictionary. Besides this, you need to join multiple datasets, check for their correctness, and develop basic summary reports.
Transform the variables:
When your data is in the right shape, you can check the distributed variables and features of univariate analysis. In addition to this, you can check relationships of other variables as well as dependent variables for multivariate analysis. You can transform the model with one of the approaches from binding approach, transformation, extreme value treatments, missing value treatment, and dimension reduction.
Select the right predictive modelling process
There are different types of predictive models. On the basis of the goals defined, you can choose one or a combination of multiple predictive modelling techniques.
Validate models through testing
Once you have selected the right predictive modelling process, you can score as well as predict test models with the help of a test sample. You can validate the robustness as well as the stability of the model and even test the model performance such as GINI, AUC, KS, ROC, and more.
What is the Future of Predictive Modelling?
With Artificial Intelligence walking multiple dimensions all at once, we can be definite about the future of predictive modelling. The computation probability will always be awaiting breakthrough as apps and enterprise solutions for eCommerce, retail, automotive, and others are witnessing an exponential rise in the data volumes, which engenders high-level complexity.
Predictive modelling will decrease data silos and reduce the risk of data security. The security encircling the data privacy when these sets are exposed can be tackled when companies and enterprises bring predictive analytics into their mainstream operations. Though what we might predict and see in actuality may be different in nature. However, it is a surefire way to avoid as many risks as possible and get to the desired outcomes ASAP.
Our data scientists tap into the power of your data to help you harness insights and eventually back you up with actionable data.